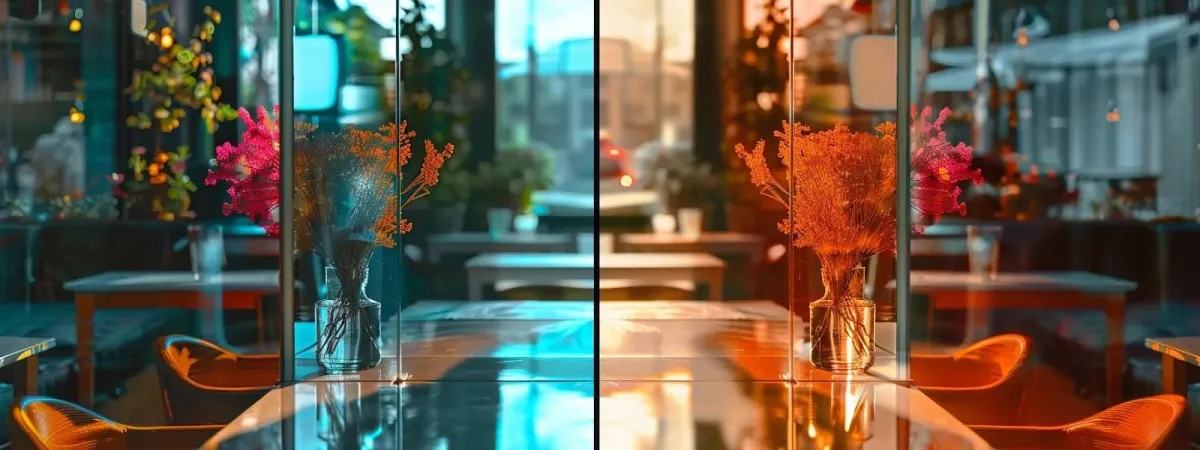
A/B Testing Best Practices for Enhancing Website Performance
Are you leveraging A/B testing to its fullest potential to enhance your website's performance? This blog post will shed light on the best practices for A/B testing, focusing on establishing clear goals, selecting pivotal elements for testing, and utilizing analytics tools effectively. You will learn how to refine your approach by interpreting test results to make informed decisions and iteratively improve your strategies. Specifically, we'll spotlight how tools like Google Optimize can tailor your content to resonate more deeply with your target audience. By integrating qualitative research into your analysis, you'll be equipped to tackle common challenges and elevate your website's success.
Understanding the Essentials of a/B Testing
At the heart of enhancing website performance lies A/B testing, an indispensable tool for refining usability testing and copywriting. Implementing A/B tests can lead to insightful research outcomes, improved customer satisfaction, and better decision-making. While multivariate testing extends the granular analysis, understanding A/B testing fundamentals is crucial. Key concepts include control groups, variables, and conversion metrics. These practices not only help in optimizing content but also enhance user experiences. This section will examine A/B testing's benefits, essential terminology, and the significant impact it has on website optimization.
What a/B Testing Is and How It Enhances Website Performance
A/B testing, at its core, is a straightforward approach to web analytics that involves presenting two variants of a web page to different segments of website visitors at the same time and comparing which variation drives better conversion rates. This method tests a hypothesis about two web page designs or content strategies to determine which one better resonates with the audience, thereby enhancing the site's value proposition. By systematically and incrementally testing hypotheses, businesses are able to refine their web presence, leading to measurable improvements in user engagement and sales conversions.
Incorporating automation into A/B testing allows for real-time data collection and analysis, streamlining the decision-making process and reducing the likelihood of errors. For instance, when experimenting with different pricing strategies, automated A/B testing can provide quick feedback on which price point maximizes profit without deterring customers. This hands-on, empirical approach to optimizing web pages cultivates a deeper understanding of consumer behavior, ultimately driving performance and creating a more effective online platform for businesses.
The Benefits of Implementing a/B Tests on Your Website
Introducing A/B testing into website development provides pivotal insights that can significantly uplift user interaction with the interface. By leveraging algorithm-powered website analytics tools, site owners can systematically evaluate the performance of different web elements through split URL testing, yielding a high-impact strategy for fine-tuning user experience. This methodical approach not only sharpens the on-page components but can also contribute significantly to achieving peak conversion rates.
Another tangible advantage of A/B testing is the effectiveness in optimizing the allocation of traffic through sampling, ensuring that the most compelling content or design features are showcased to visitors. As businesses strive to understand user preferences, these tests unveil invaluable data, allowing for iterative enhancements that resonate with the target audience. The strategic application of A/B testing accelerates the evolution of a website, making it more adaptable and attuned to the dynamic needs of the market.
Key Concepts and Terminology in a/B Testing
A/B testing is a refined technique that involves comparing different versions of a user interface or web page to determine which variation performs better in terms of user engagement and conversion rate optimization. Central to this practice is the understanding of the 'funnel,' a concept that represents the journey a user takes from landing on a website to completing a desired action. By scrutinizing how alterations to the interface influence user behavior at various stages of the funnel, businesses can harness data-driven insights to heighten their website's effectiveness.
The utilization of machine learning in A/B testing has emerged as a modern approach that greatly amplifies the potential to strategically enhance user experience. Machine learning algorithms can predict and analyze user attention patterns, thereby identifying the most impactful elements in user interface design. This foresight allows for targeted experiments that directly address areas of a web page most likely to influence the visitor's decision-making process, facilitating a more sophisticated form of conversion rate optimization.
Establishing Clear Goals and Hypotheses
Setting specific objectives and crafting data-driven hypotheses are foundational steps in A/B testing that lead to impactful website optimization. When tests are strategically prioritized based on their potential to affect user preference and innovation in usability, the process becomes significantly more efficient. Incorporating Bayesian statistics elevates the confidence in testing outcomes, offering a methodical approach to enhancing user experiences. The subsequent sections will delve into these practices, showcasing their crucial role in refining web performance through targeted experimentation.
Setting Specific Objectives for Your a/B Tests
Establishing specific and measurable objectives is central to the success of any A/B testing initiative as it directly impacts content management strategies. When tests are crafted with precision, focusing on aspects such as customer success and homepage optimization, businesses can make informed decisions that yield a higher return on investment. For instance, improving call-to-action button visibility may directly correlate with increased customer conversion rates, offering direct insight into user behavior and preferences.
During the experiment, it's crucial to align goals with quantitative data points to effectively measure outcomes. If the objective is to enhance homepage engagement, metrics such as session length and bounce rate can reveal how modifications affect user interaction. Setting these clear targets for each experiment ensures that every variation tested provides actionable data and drives the company's content management process forward to ensure a positive impact on overall website performance.
Crafting Data-Driven Hypotheses to Guide Testing
Developing data-driven hypotheses requires understanding the variables that significantly impact user behavior and, ultimately, revenue. A practical hypothesis may focus on how altering a single parameter, such as button color or placement, can lead to increased customer interaction on a website. Efficient use of a testing tool can assist in predicting the probability of different outcomes, ensuring hypotheses are not just educated guesses but grounded in past user engagement data.
For meaningful A/B testing, it's essential to formulate hypotheses that are directly linked to measurable outcomes. For instance, a hypothesis might assert that rearranging the layout of a product page will enhance the user experience, resulting in a higher probability of purchase and increased revenue. This assertion sets a clear direction for the testing process and allows for the collection of targeted, actionable data, which can then inform subsequent optimization strategies.
Prioritizing Tests Based on Potential Impact
Prioritizing A/B tests according to their potential impact involves strategic data analysis to foresee which changes will most effectively drive conversions and user satisfaction. For instance, using tools like Unbounce for landing page optimization can help identify key behaviors that, when adjusted, predict a higher likelihood of user engagement. This targeted approach ensures that more resources are allocated to high-value modifications that are proven to benefit a site's metrics and user experience.
In the ever-evolving landscape of mobile technology, testing mobile apps must also be approached with a keen eye for user behavior prediction. By focusing on how different design choices or feature implementations affect app usability and appeal, businesses can employ A/B tests to refine their mobile presence. Keeping the user's behavior at the forefront of analysis allows for optimizing the mobile experience, which is pivotal for staying competitive in a market that increasingly prioritizes handheld device compatibility.
Selecting the Right Elements to Test for Maximum Results
Choosing the right elements to test is pivotal in maximizing the effectiveness of A/B testing strategies. Identifying high-impact components for experimentation, such as key conversion points on a website, directly influences the customer experience. When tests focus on singular variables, they yield clearer insights, ensuring results reflect actual user responses rather than confounding factors. The selection of relevant metrics is equally important, as it defines the baseline for measuring performance and achieving statistical significance. This subsection will provide an understanding of how using the right testing tools and grounding decisions in data rather than opinion leads to a more compelling website performance.
Identifying High-Impact Website Components to Experiment With
When sharpening the focus of A/B testing, it's crucial to pinpoint components on your website that will most significantly impact user behavior. For example, testing the onboarding process on a mobile app can provide valuable insights into reducing the learning curve and improving user retention. Similarly, employing Adobe Analytics to examine variations on a landing page allows for precise tracking of user engagement and identifiying which version facilitates a smoother conversion path.
Experimentation with elements that have a high probability of affecting user decisions can transform the effectiveness of a website. Landing page testing often yields actionable results by revealing the strengths and weaknesses of page design, content, and calls-to-action. By analyzing the data collected through Adobe Analytics, site owners can understand the user's journey, making informed decisions that refine the onboarding experience, streamline user flow, and enhance the overall performance of both web and mobile app platforms.
Focusing on Single Variables to Ensure Accurate Results
In the nuanced world of conversion marketing, isolating single variables is a statistical imperative that ensures test results are attributable to the change made, rather than external factors. For example, when evaluating the user experience, changing one element such as the color or text of a button allows site owners to draw clear conclusions on its impact. This precision avoids the confounding effects that can come from multi-variable changes and strengthens the validity of the A/B test findings.
Insights gained from focusing on individual elements have a profound effect on user interaction and customer support. When a business tests a new live chat prompt button, centering the experiment on the button's position alone offers clear data on optimal placement for user engagement. This targeted approach to testing simplifies decision-making and provides a robust framework for understanding how small changes can significantly influence customer behavior on the site.
Choosing Relevant Metrics to Measure Performance
Selecting the most relevant metrics to gauge A/B testing performance is critical for obtaining meaningful insights into user behavior. When refining a page layout or placing a call to action, it's important to monitor metrics like click-through rates and conversion percentages. These numbers reflect direct user interaction, allowing businesses to assess the efficacy of changes made to their website's design and content personalization efforts.
For businesses looking to optimize their websites for search engines while improving user experience, observing changes in organic search traffic can reveal the impact of A/B testing. By focusing on how adjustments to elements such as personalization strategies or page layout influence not only user decisions but also search engine visibility, companies can implement data-driven design and content improvements that drive both engagement and search engine ranking.
Implementing a/B Tests Effectively
To effectively enhance website performance through A/B testing, employing reliable tools such as Optimizely or VWO is crucial for creating robust landing page experiments. Determining the right sample sizes and test durations minimizes risk, ensuring statistical significance and accurate results. Additionally, maintaining a seamless user experience during tests is essential, so that the data reflects genuine user behavior unaffected by any interruptions or glitches. The following sections will delve into best practices for using these tools, setting up tests properly, and keeping the user experience intact throughout the process.
Utilizing Reliable Testing Tools and Platforms
Choosing the right A/B testing platform is crucial for gaining accurate insights into customer service improvements and maximizing lead generation. Tools with advanced features like session replay facilitate a deeper understanding of the visitor experience, allowing businesses to observe user interactions in real-time and pinpoint areas for enhancement. This can streamline the workflow, enabling teams to implement changes that directly contribute to a more effective and intuitive customer journey on the website.
Reliable A/B testing software provides a solid framework for conducting controlled experiments and gathering actionable data. These platforms can help businesses to refine their lead generation strategy by correlating specific website changes with user behavior. Utilizing platforms that offer robust analytics and session replay can lead to significant optimizations in the customer service workflow, ultimately translating into improved conversion rates and a healthier bottom line for businesses.
Determining Optimal Sample Sizes and Test Durations
Choosing the appropriate sample size for A/B tests is a critical aspect of quantitative research pivotal to the accuracy of data collection. A sample too small may not capture the full spectrum of user behavior, leading to inconclusive results, while an excessively large sample could squander resources. The sweet spot lies in a sample size that reflects the diversity of website visitors and accounts for variables that might affect motivation and bounce rate, thus providing reliable data for informed decision-making.
As for the duration of A/B tests, balance is key to ensure enough data is collected without prolonging the test unnecessarily. Short tests may provide a snapshot of user behavior but risk overlooking long-term trends and changes in motivation, whereas tests that are too long could lead to stale data as market conditions evolve. An optimal test duration allows for observing user interactions across a complete sales cycle, giving a comprehensive view of how changes affect metrics like bounce rate and overall user behavior.
Ensuring a Smooth User Experience During Testing
When conducting A/B tests, it is paramount that user navigation remains intuitive and consistent to avoid skewing data with frustrating user experiences. Effective A/B tests should integrate seamlessly with the site's overall optimization strategy, taking care not to disrupt the user's scroll path or conversion funnel. This attention to maintaining a coherent journey ensures that the data collected on user behavior is reflective of actual preferences, not reactions to test-induced confusion.
In the realm of content marketing, the impact of A/B testing is largely determined by how inconspicuously these tests are implemented. For testers, the goal is to subtly alter elements without reducing the quality or accessibility of the content that users have come to expect. Such meticulous optimization efforts during A/B testing contribute not only to the clarity and satisfaction within the user experience but also to more reliable data that informs future digital strategies.
Analyzing Results and Making Informed Decisions
After implementing A/B testing, the critical phase of interpreting test results begins. Analyzing these results with statistical significance is key to unlocking the intelligence behind your data, guiding you toward smarter conversion optimization strategies. Extracting actionable insights from test outcomes empowers you with the knowledge needed to refine and enhance website performance comprehensively. The forthcoming sections will detail the process of sifting through analytics, interpreting their significance, and applying findings to solidify your website's effectiveness.
Interpreting Data With Statistical Significance
Discerning the statistical significance of A/B test results is foundational to validating the reliability of changes made to a web page. This rigorous analysis ensures that the data observed from altering a site's marketing strategy reflects actual performance improvements and not random variation. Tools like ContentSquare can provide an in-depth look at user interactions, bolstering the confidence that a shift in the website's proposition is genuinely resonating with the audience.
When interpreting A/B testing outcomes, marketers must determine if the observed differences in user behavior are statistically substantial enough to inform future web development decisions. This entails a deep dive into metrics and probabilities, ensuring the chosen marketing strategy is built on concrete evidence rather than conjecture. Accurate interpretation of these statistics lets businesses refine their web page layouts and propositions in a way that profoundly impacts user engagement and conversion rates.
Extracting Actionable Insights From Test Outcomes
After concluding an A/B test, distilling actionable insights from the data is where the true value of the testing process is realized. It's essential to discern patterns and anomalies in the results, which can provide a roadmap for iterative improvement. For example, if a particular call-to-action led to an uptick in sign-ups, this data point might suggest a user preference for language, color, or placement, guiding future design choices.
Translating test outcomes into strategic actions requires a nuanced understanding of metrics and user behavior. Scrutinizing details like click distribution or time spent on a page after an adjustment can reveal subtle user preferences. These insights lead to refined tactics, such as tweaking content structure or simplifying navigation, to not only meet but exceed user expectations and drive website performance.
Applying Findings to Enhance Website Performance
Transforming A/B test findings into performance enhancements requires a strategic approach to website optimization. By interpreting the data with precision, businesses can pinpoint the exact changes that lead to better user engagement and conversion rates. Utilizing tools for in-depth analytics allows for the application of these insights into practical steps that improve the website's architecture and design, ensuring each tweak aligns with consumer behavior and preferences.
Once conclusive data highlights a successful variation in an A/B test, it's critical for businesses to implement these changes consistently across the website. This aligns the user experience with tested preferences, creating a cohesive and optimized online environment. By systematically applying these results, businesses ensure that the enhancements contribute positively to the site's overall performance, solidifying the link between data-driven decisions and improved user interaction.
Continuously Improving Through Iterative Testing
Mastering A/B testing is a dynamic process that demands a robust, forward-thinking strategy. This entails not only formulating a systematic approach to long-term testing but also distilling knowledge from past experiments to sharpen future ones. Scaling successful modifications site-wide is the ultimate goal, ensuring that incremental gains in performance are broadly applied. The upcoming discussion will delve into these critical facets of A/B testing, highlighting the path to continuous improvement and optimal website efficiency through strategic experimentation.
Developing a Long-Term a/B Testing Strategy
Establishing a long-term A/B testing strategy enables continuous learning and refinement of a website's performance. It involves a cycle of testing, analyzing, learning, and applying insights to form an iterative process that steadily enhances user experience and conversion rates. A structured approach, grounded in clear objectives and informed by past test outcomes, allows businesses to remain agile in responding to evolving user behaviors and market conditions.
In developing this kind of testing strategy, it's vital to build upon the data acumulated from ongoing experiments. Regular analysis of this data helps in recognizing patterns and trends that shape more informed hypotheses for future tests. By committing to this ongoing process, companies can foster a culture of data-driven decision-making that perpetually optimizes the website's elements, ensuring optimal performance and pronounced user satisfaction.
Learning From Past Tests to Refine Future Experiments
Extracting lessons from past A/B tests is instrumental in refining the hypothesis for subsequent experiments. Continuous analysis of user behavior, conversion rates, and other performance indicators from previous A/B tests informs data-driven decisions. This retrospective insight ensures that future tests are more finely tuned and apt to uncover deeper nuances of user experience, paving the way for more effective website enhancements.
By evaluating what has been successful and identifying areas for improvement, teams can develop a keen eye for detail that prevents the repetition of ineffective strategies. An organization's commitment to learning from each testing iteration shapes an evolving understanding of its audience. This evolution translates into enhanced website performance through an iterative process of experimenting, learning, and applying, all shaped by the informative power of past A/B testing outcomes.
Scaling Successful Changes Across Your Website
Scaling successful A/B test changes across a website is akin to solidifying a winning strategy into the digital DNA of a brand's online presence. Once data has proven a certain change improves user engagement or conversion rates, expanding this alteration site-wide is crucial. This consistent application of proven enhancements contributes to a seamless user experience, reinforcing positive behavior patterns and solidifying the gains achieved through meticulous testing.
Applying successful A/B testing outcomes comprehensively ensures that all visitors encounter the optimized elements, thereby uniforming the enhanced experience. It is essential that these updates are rolled out methodically to prevent any disruption and to maintain the integrity of the data upon which the changes were based. Through careful execution, businesses can assure that their website reflects the latest in user experience optimization, driving overall performance and satisfaction in a significant, measurable way.
Conclusion
A/B testing stands as a paramount process in fine-tuning website performance, constantly informed by rigorous data analysis and user behavior insights. Through diligent implementation of tests focused on single variables and utilization of reliable analytics tools, businesses can glean actionable insights to drive user engagement and conversion. Beyond isolated improvements, the strategic application of successful A/B test findings across a website manifests a cohesive and optimized user experience. Continuously refining this cycle crystallizes A/B testing not just as a practice, but as a cornerstone of ongoing digital success and customer satisfaction.
Facebook
Instagram